Project Introduction
Official microdata related Project
Project of Synthesis Credit Risk Database Consortium
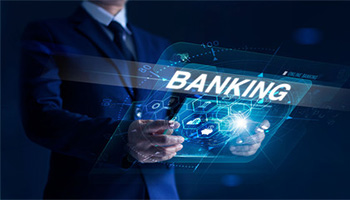
Project Summary and Anticipated Outcomes and Goals
This study deals with the estimation of expected loss using a Synthesis Credit Risk Database of five banks. This includes information on collateral, guarantees, and debt collection, which is not publicly available. Based on this data, the study develops and proposes a versatile and precise estimation method.
Thus, a method for the practical use of statistical and machine learning approaches in the estimation of expected loss has been established. The results will be used to help develop credit risk research, improve the sophistication of banks’ loan screening, streamline financial administration, and facilitate small and medium-sized enterprise finance.
Project Background
Banks play an important role in indirect finance. Since financial crisis, they have been given the urgent task of creating a more effective their risk management measures than the existing one. In addition to the lending risk faced by banks, the estimated loss of the companies in the lender’s portfolio is a problem. The factors in expected loss can be categorized as Probability of Default (PD) and Loss Given Default (LGD). The estimate of LGD, particularly, is an important indicator for the BASEL II Advanced Internal Ratings-Based Approach (new BIS regulations) and when calculating loan loss reserves under International Financial Reporting Standards (IFRS). However, as a result of the limited integrated database, only a few methods have been proposed for the LGD estimation (Chart-1).
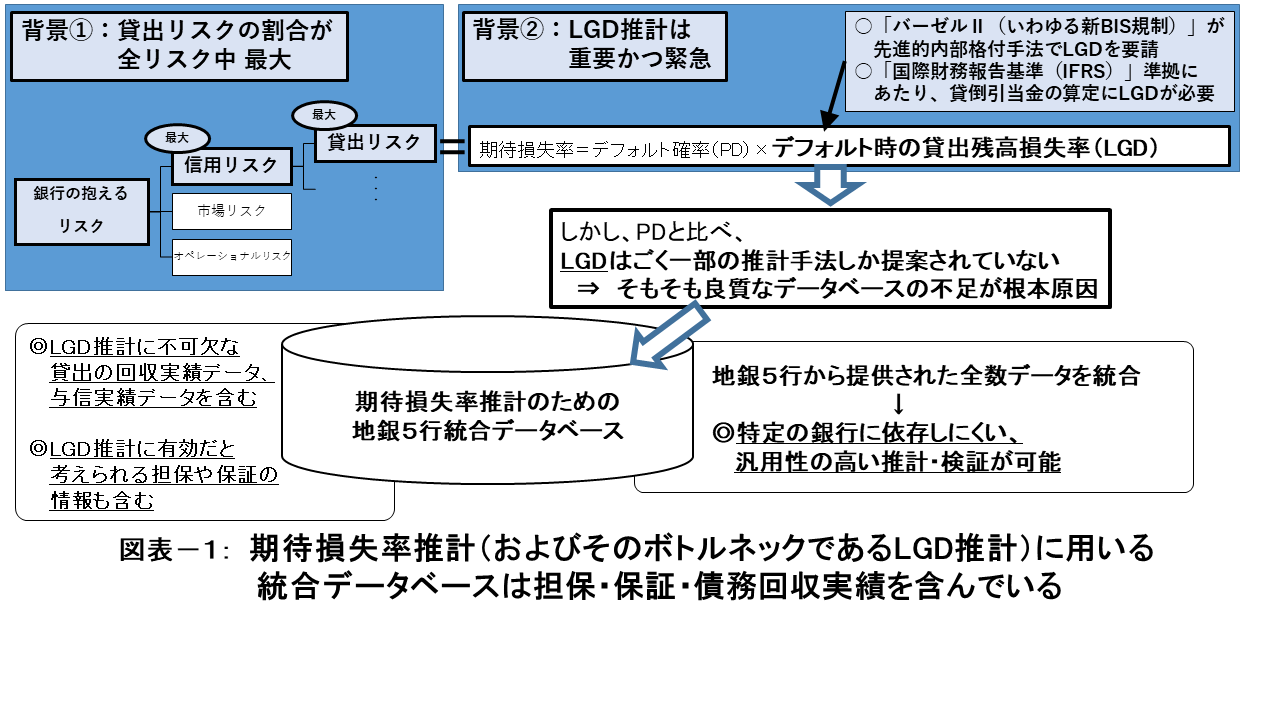
Project Research and Development Content
As evidenced by the 2008 financial crisis, more elaborate risk management by banks is required for the stability of finance and economics in society. The risk held by banks can be broadly divided into three types: credit risk, market risk, and operational risk. The greatest risk out of these three is credit risk, and among credit risk, risk on lending is known to be the major risk faced by banks.
Lending risk is mainly supplemented by the expected loss of the lender. This can be broadly divided as (1) Probability of Default (PD), and (2) Loss Given Default (LGD). PD is the probability that a lender will default, that is, that they will fail to make repayment within a certain period (usually a year).
LGD describes the amount of loans outstanding at the time of default as a ratio of the actual loss of the defaulting lender. Banks should make precise estimates of these factors for accurately assessing the risk of loan assets.
Basel II is a new type of regulation on capital adequacy that aims to maintain the soundness of internationally operating banks. It states that credit risk should be estimated using (A) a standardized method (via external ratings) and (B) an internal ratings method. The internal ratings method (B) can be classified as (B-1) “Fundamental Internal Ratings-Based Approach,” which estimates only the PD, and (B-2) “Advanced Internal Ratings-Based Approach,” which estimates both the PD and LGD.
However, the lack of an integrated database due to the highly confidential nature of bank data poses difficulties in estimating the credit risk of bank assets using the aforementioned method. In particular, the number of databases for LGD estimation, including (1) “actual loan collections” (figures on actual collections, that is, incurred losses by banks through their lending), which is essential for LGD analysis, is severely lacking.
Furthermore, the practical use of highly accurate LGD estimation methods requires (2) the inclusion of variables that are considered effective, such as “collateral” and “guarantees,” and (3) the existence of a database that can integrate data from multiple banks so that the results are not bank-specific. The Synthesis Credit Risk Database Consortium was built for the purpose of creating a single database for evaluation of credit risk in Japan that satisfies conditions (1) through (3). The Synthesis Credit Risk Database of five banks was created for the estimation of expected loss using this research.
The following subthemes are being elucidated to build an optimal estimation model using statistical models and machine learning in the integrated database.
(a) Compliance with BASEL II/IFRS
We are performing a comprehensive review on cutting edge statistical models and machine learning model based estimation accuracy standards.
(b) Model with the highest estimation accuracy
We are performing a comprehensive review on cutting edge statistical models and machine learning model based estimation accuracy standards.
(ii) Comprehensive review of cutting-edge models and machine learning models
To find the optimal variables for a model, we are using variable selection methods based on mathematical statistics, methods checked from the operator’s perspective, and comprehensive and exhaustive methods that fully utilize computational resources during the exploration process.
(d) Optimal parameters for the model
We are using methods such as least squares estimation, maximum likelihood estimation, and Bayesian estimation to obtain the optimal parameters.